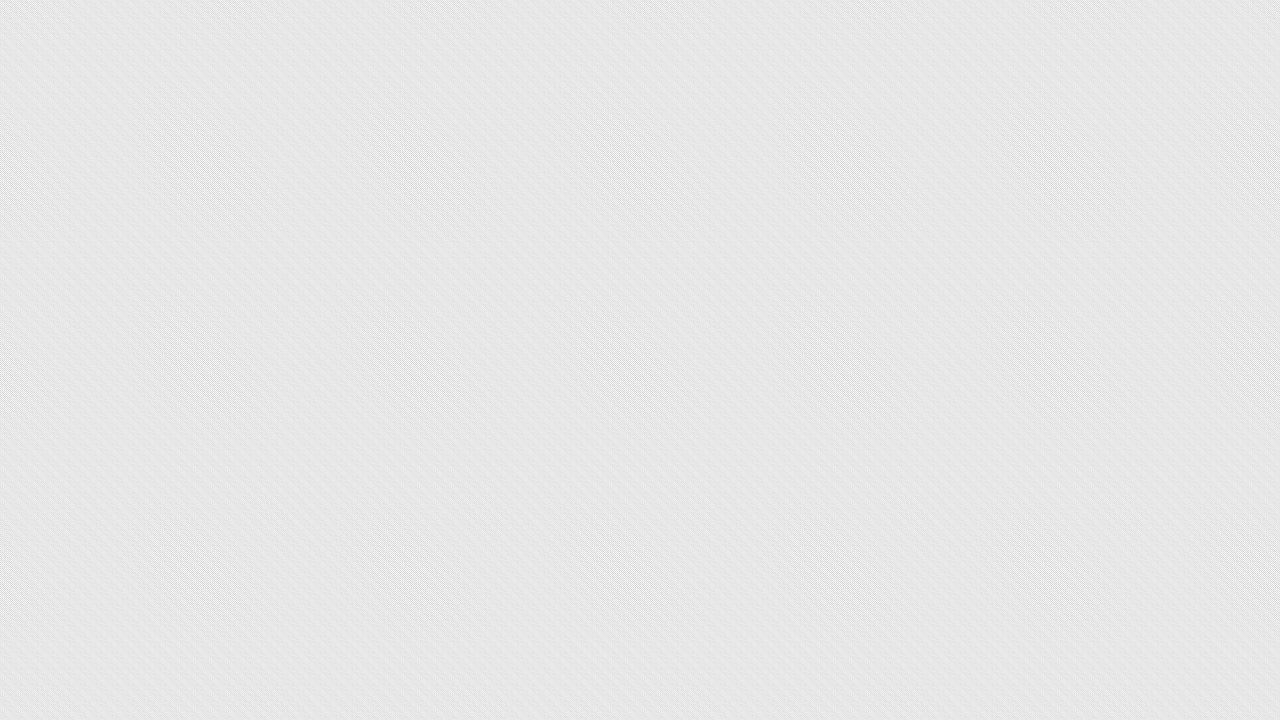
Research Highlights
-
Formal statistical methods for large or massive spatial and spatio-temporal data.
​
-
Bayesian methodology for uncertainty quantification and computer experiments.
​
-
Computational statistics for high-dimensional data analysis, graphical models, and network analysis.
​
-
Providing data analytic solutions for problems across climate, engineering, biological sciences and econometrics.
Publications (* denotes advisees/students in GDADS when the research was conducted.)
​
Note: Software tools posted below are based on the methodological and computational research done by GDADS. They can be used for non-commercial purposes without restriction. We kindly ask you to acknowledge, cite, and/or reference relevant GDADS publications.
Collaborative work​
​
-
Ojiambo, P. S. and Kang, E. L. (2013) Modeling spatial frailties in survival analysis of cucurbit downy mildew epidemics. Phytopathology, 103, 216-227. [Link]
-
Ren, S.*, Hinzman, A. A., Kang, E. L., Szczesniak, R. D., and Lu, L. J. (2015) Computational and statistical analysis of metabolomics data. Metabolomics, 11, 1492-1513, doi: 10.1007/s11306-015-0823-6. [Link]
-
Huang, Y., Liu, H., Yu, B., Wu, J., Kang, E. L., Xu, M., Wang, S., Klein, A., and Chen, Y. (2018) Improving MODIS snow products with a HMRF-based spatio-temporal modeling technique in the Upper Rio Grande Basin. Remote Sensing of Environment, 204, 568-582. [Link]
-
Xu, M, Liu, H., Beck, R., Lekki, J., Yang, B., Shu, S., Kang, E. L., Anderson, R., Johansen, R., Emery, E., Reif, M., and Benko, T. (2018) A spectral space partition guided ensemble method for retrieving chlorophyll-a concentration in inland waters from Sentinel-2A satellite imagery. Journal of Great Lakes Research, https://doi.org/10.1016/j.jglr.2018.09.002. [Link]
-
Cawse-Nicholson, K., Fisher, J. B., Famiglietti, C. A., Braverman, A., Schwandner, F. M., Lewicki, J. L., Townsend, P. A., Schimel, D. S., Pavlick, R., Bormann, K. J., Ferraz, A., Kang, E. L., Ma, P.*, Bogue, R. R., Youmans, T., and Pieri, D. C. (2018) Ecosystem responses to elevated CO2 using airborne remote sensing at Mammoth Mountain, California. Biogeosciences, 15, 7403-7418, doi: 10.5194/bg-15-7403-2018. [Link]
-
Zhu, Y., Kang, E. L., Bo, Y., Zhang, J., Wang Y., and Tang, Q. (2019) Hierarchical Bayesian model based on robust fixed rank filter for fusing MODIS SST and AMSR-E SST. Photogrammetric Engineering & Remote Sensing, 85, 119-131. [Link] [pdf]
-
Liu, L., Lan, M., Eck, J. E., and Kang, E. L. (2020) Assessing the effects of bus stop relocation on street robbery. Computers. Environment and Urban Systems, 808, 101455. [Link]
-
Cawse-Nicholson, K., Braverman, A., Kang, E. L., Li, M.*, Johnson, M., Halverson, G., Anderson, M., Hain, C., Gunson, M., and Hook, S. (2020) Sensitivity and uncertainty quantification for the ECOSTRESS evapotranspiration algorithm - DisALEXI. International Journal of Applied Earth Observation and Geoinformation, in press. [Link]
-
Shu, S., Liu, H., Frappart, F., Kang, E. L., Yang, B., Xu, M., Huang, Y., Wu, B., Yu, B. Wang, S., Beck, R., and Hinkel, K. (2020) Improving Satellite waveform altimetry measurements with a probabilistic relaxation algorithm. IEEE Transactions on Geoscience and Remote Sensing, in press. [Link]
​
Methodology
​
-
Kang, E. L., Liu, D., and Cressie, N. (2009). Statistical analysis of small-area data based on independence, spatial, non-hierarchical, and hierarchical models. Computational Statistics and Data Analysis, 53, 3016-3032. [Link]
-
Morton, R., Kang, E. L., and Henderson, B. (2009). Smoothing splines for trend estimation and prediction in time series. Environmetrics, 20, 249-259. [Link]
-
Cressie, N. and Kang, E. L. (2010). High-resolution digital soil mapping: Kriging for very large datasets. In Proximal Soil Sensing, eds R. Viscarra-Rossel, A. B. McBratney, and B. Minasny. Springer, Dordrecht, 49-63. [Link]
-
Cressie, N., Shi, T., and Kang, E. L. (2010). Fixed rank filtering for spatio-temporal data. Journal of Computational and Graphical Statistics, 19, 724-745. [Link] [Matlab Code] *The supplementary material at the journal website is incomplete.
-
Kang, E. L., Cressie, N., and Shi, T. (2010). Using temporal variability to improve spatial mapping with application to satellite data. Canadian Journal of Statistics, 38, 271-289. [Link]
-
Kang, E. L. and Cressie, N. (2011). Bayesian inference for the spatial random effects model. Journal of the American Statistical Association - Theory and Methods, 106, 975-983. [Link]
-
Kang, E. L., Cressie, N., and Sain, S. R. (2012). Combining outputs from the North American Regional Climate Change Assessment Program by using a Bayesian hierarchical model. Journal of the Royal Statistical Society, Series C (Applied Statistics), 61, 292-313. [Link]
-
Kang, E. L. and Harlim, J. (2012). Filtering nonlinear spatio-temporal chaos with autoregressive linear stochastic model. Physica D, 241, 1099-1113. [Link]
-
Kang, E. L. and Harlim, J. (2012). Filtering partially observed multiscale systems with Heterogeneous Multiscale Methods based reduced climate models. Monthly Weather Review, 140, 860-873. [Link]
-
Kang, E. L. and Cressie, N. (2013) Bayesian hierarchical ANOVA of regional climate change projections from NARCCAP Phase II. International Journal of Applied Earth Observation and Geoinformation, 22, 3-15. [Link]
-
Kang, E. L., Harlim, J., and Majda, A. J. (2013) Regression models with memory for the linear response of turbulent dynamical systems. Communications in Mathematical Sciences, 11, 481-498. [Link]
-
Cressie, N., and Kang, E. L. (2015) Hot enough for you? A spatial exploratory and inferential analysis of North American climate-change projections. Mathematical Geosciences, 48, 107-121, doi:10.1007/s11004-015-9607-9. [Link]
-
Zhu, Y., Kang, E. L., Bo, Y., Tang, Q., Cheng, J., and He, Y. (2015) A robust Fixed Rank Kriging method for Improving the spatial completeness and accuracy of satellite SST products. IEEE Transactions on Geoscience and Remote Sensing, 53, 5021-5035. [Link]
-
Shi, H.* and Kang, E. L. (2017) Spatial data fusion for large non-Gaussian remote-sensing datasets. Stat, 6, 390-404, doi:10.1002/sta4.165 [Link] [Supplementary] [Matlab Code]
-
Shi, H.*, Kang, E. L., Konomi, B. A., Vemaganti, K., and Madireddy, S. (2017) Uncertainty quantification using the nearest neighbor Gaussian process. In D.G. Chen, Z. Jin, G. Li, Y. Li, A. Liu, Y. Zhao (Editors), New Advances in Statistics and Data Science, ICSA Book Series in Statistics. Springer, 89-107. [Link]
-
Ma, P.*, Kang, E. L., Bravermen, A., and Nguyen, H. (2018) Spatial statistical downscaling for constructing high-resolution nature runs in global observing system simulation experiments. Technometrics, doi: 10.1080/00401706.2018.1524791. [Link]
-
Li, M.* and Kang, E. L. (2019) Randomized algorithms of maximum likelihood estimation with spatial autoregressive models for large-scale networks. Statistics and Computing, 29, 1165-1179, doi: 10.1007/s11222-019-09862-4. [Link]
-
Ma, P.*, Konomi, B. A. and Kang, E. L. (2019) An additive approximate Gaussian process for large spatio-temporal data. Environmetrics, https://doi.org/10.1002/env.2569. [Link]
-
Konomi, B. A., Hanandeh, A. A.*, Ma, P.*, and Kang, E. L. (2019) Computationally efficient nonstationary nearest neighbor Gaussian process models using data-driven techniques. Environmetrics, https://doi.org/10.1002/env.2571. [Link]
-
Ren, S.*, Kang, E. L., and Lu, J. L. (2020) MCEN: a method of simultaneous variable selection and clustering for high dimensional multinomial regression. Statistics and Computing, https://doi.org/10.1007/s11222-019-09880-2. [Link]
-
Ma, P.* and Kang, E. L. (2020) Spatio-temporal data fusion for massive sea surface temperature data from MODIS and AMSR-E instruments. Environmetrics, https://doi.org/10.1002/env.2594. [Link]
-
Ma, P.* and Kang, E. L. (2020) A fused Gaussian process for very large spatial data, Journal of Computational and Graphical Statistics, forthcoming. [Link]
-
Stough, T., Cressie, N., Kang, E. L., Michalak, A. M., and Sahr, H. (2020) Spatial analysis and visualization of global data on multi-resolutional hexagonal grids, Japanese Journal of Statistics and Data Science, forthcoming. [Link]
-
Yang, B., Liu, H., Kang, E. L., Shu, S., Xu, M., Wu, B., Beck, R. A., Hinkel, K., and Yu, B. (2021) Spatio-temporal cokriging method for assimilating and downscaling multiscale remote sensing data, Remote Sensing of Environment, forthcoming. [Link]
-
Ma, P., Mondal, A., Konomi, B. A., Hobbs, J., Song, J. and Kang, E. L. (2021+) Computer model emulation with high-dimensional functional output in large-scale observation system uncertainty experiments, Technometrics, forthcoming.
​
Non-refereed work ​
​
-
Cressie, N. and Kang, E. L. (2008). Soil mapping using spatial statistics: Kriging for very large datasets. In Proceedings of First Global Workshop on High Resolution Digital Soil Sensing and Mapping, Vol. I, Sydney, Australia (14pp.).
-
Gilleland, E., Chen, L., DePersio, M., Do, G., Eilertson, K., Jin, Y., Kang, E. L., Lindgren, F., Lindstr ̈om, J., Smith, R. L., and Xia, C. (2011). Spatial Forecast Verification: Image Warping. NCAR Technical Notes, TN-482+STR, 30pp. [pdf]
​